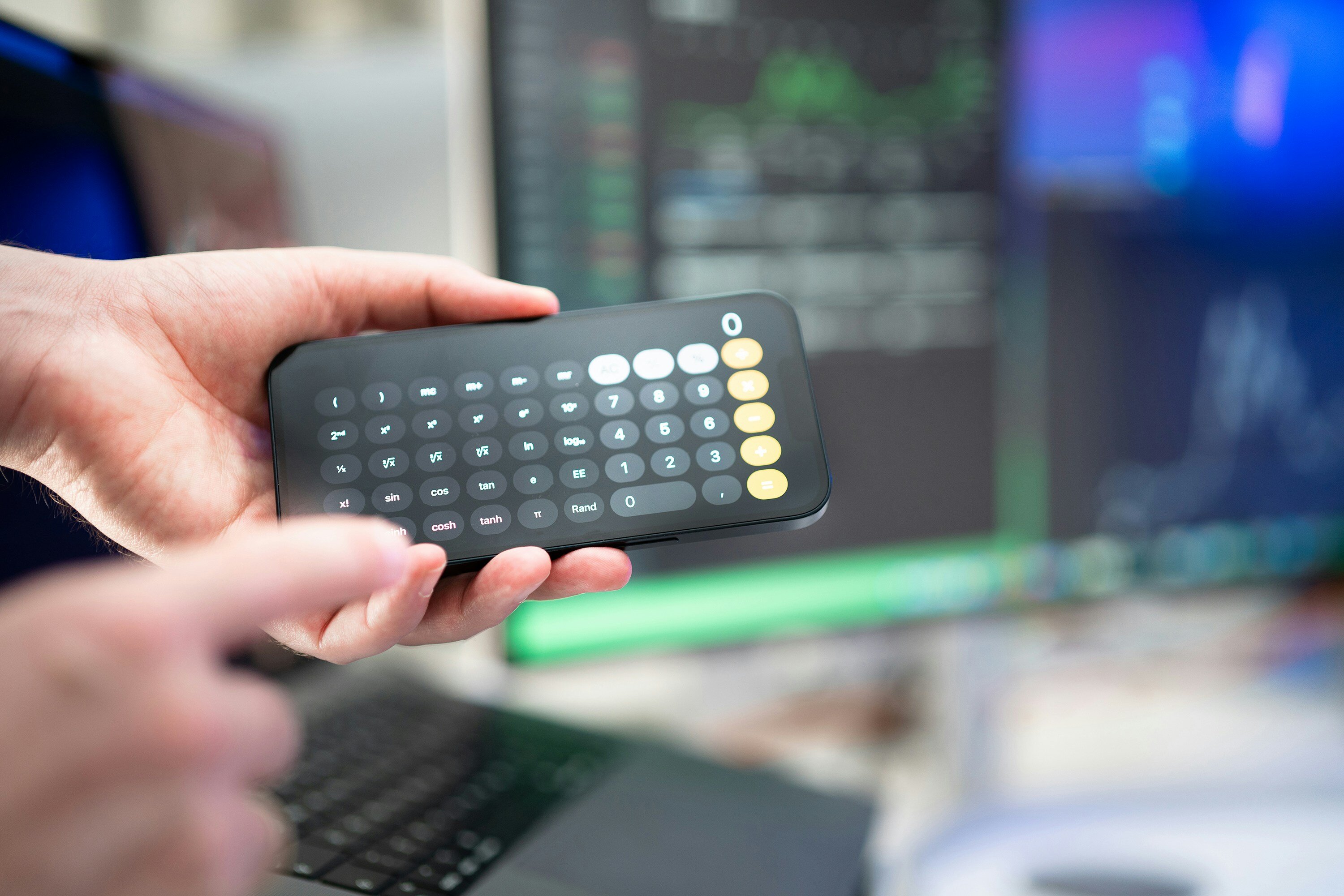
Show Me Your Back Test and I Show You Your Risk
Learn how to leverage Quantitative Investment Strategies (QIS) backtests to understand risks, despite their limitations in predicting future performance. Discover the potential and challenges of QIS.
7 min read | Dec 4, 2024
In a series of previous posts, we highlighted the huge potential of Quantitative Investment Strategies (QIS) for creating profitable and risk-controlled portfolios. QIS are systematic absolute return strategies calculated as indices and offered by investment banks. In this sense, QIS could be seen as the “ETFs for absolute return strategies,” offering higher liquidity, greater transparency, and often lower costs compared to hedge fund investments.
The Challenges of Assessing New Strategies
Today, QIS represent a vast market. At Resonanz Capital, we currently track more than 2,000 different indices across 11 investment banks. Each bank has its own teams focusing on R&D for new strategies. In a previous post, we demonstrated the huge potential of “brain diversification”—investing with multiple investment banks leverages the wisdom of these R&D teams to achieve very attractive risk-adjusted returns. While this market proliferation offers immense potential for investors, it also presents significant challenges. Newly developed strategies often have a short live track record, if any, for investors to analyze. Investment banks typically provide investors with extensive backtesting data for these strategies. However, a backtest is not the same as a live strategy.
This raises the question: What information might be helpful in assessing the potential of a new strategy for an investor's portfolio? Answering this question is of central importance, as it will determine whether investors can benefit from new developments early on or should remain on the sideline until they better understand the game. In this post, we argue that investors should clearly ignore backtests when evaluating a strategy's profitability but might find them helpful in understanding the strategy’s risks.
Comparing Backtests and Live Performance
We use our entire universe of more than 2,000 QIS strategies to address these questions. For reliability, we include only QIS indices with at least three years of live performance. We split each strategy's track record into a backtesting period and a live period. Using these, we calculate the moments of the return distributions—e.g., annualized returns, volatility, Value at Risk (VaR), and Conditional Value at Risk (CVaR)—and compare them across the two periods.
The Bias in Backtested Returns
Let’s begin by analyzing the first moment: the annualized returns of QIS strategies. Maximizing expected returns is often the primary objective for investors. If backtests are informative about expected returns, investors could identify high-performing strategies early, before they become crowded. To assess the estimation error (or backtest bias), we calculate the annualized return for the live period and subtract the backtested annualized return for each QIS strategy. The chart below illustrates the distribution of these estimation errors:
It is striking that the distribution centers significantly below zero. On average, the backtest period overstates returns by 4.1 percentage points compared to the strategies’ live performance. Some may argue that historic returns are generally a poor proxy for future returns. If that were true, however, we would expect the estimation error distribution to center around zero, with fat tails on both sides. Instead, we find that the estimation error is negative in 86% of cases - and this is far from random, suggesting that QIS backtests systematically overstate performance.
Consistency of Backtest Bias Across Time
Some may argue that differences in market conditions between the backtest and live periods might explain these discrepancies. If that were the case, we would expect the estimation error to fluctuate over time, occasionally being positive. To test this, we plot the rolling 1-year performance of an equally weighted composite of all live and backtested strategies at each point in time:
The chart shows that the backtest composite performance consistently exceeds the live composite performance. The estimation error is persistently negative. Moreover, except for backtested hedge strategies during the COVID-19 outbreak, the backtest composite is much more stable than the live composite. We can thus conclude that backtests do indeed overstate performance.
Variations in Backtest Bias by Banks, Asset Classes and Strategy
The next logical question is whether this backtest bias varies across investment banks, strategies, or asset classes. A better understanding will help investors if there are cases where they should view bak tested more scaptical than in others.
Starting with individual banks, the chart above shows a boxplot of the estimation error grouped by bank. There is notable variation between banks. Bank 11 has the lowest median estimation error (-2.1%), while Bank 8 shows the highest (-5.4%). Additionally, the distribution width varies: some banks exhibit narrow clustering around the median, while others show very fat tails. This suggests that investors should consider the bank providing the backtest, as it might indicate how reliable the back tested performance is. Although backtested performance is generally overstated, the magnitude and dispersion of errors vary significantly by bank.
When examining asset classes, the estimation error is broadly similar across categories, except for Multi-Asset, which shows a median error of -7.5%. So we see that investors can generally apply the same discounting to backtests across asset classes. However, this does not hold at the strategy level. For example, ESG strategies exhibit a positive median error of 1.0%, as shown in the chart below and investors should carfuly asess the back tested performance depending on the strategy type.
Can Backtests Be Useful for Risk Analysis?
In summary, investors should not rely on QIS backtests to predict expected returns. But can backtests provide any useful information? Next, we examine how well higher moments, such as volatility and tail risk, are simulated. The chart below shows the distribution of the volatility estimation error for all QIS indices:
Unlike annualized returns, the volatility estimation error centers around zero, with the mean and median close to zero. This indicates no systematic bias in volatility estimates from backtests. However, investors should remain cautious, as roughly 50% of QIS backtests over- or understate realized volatility by more than one percentage point. This could either be driven by a back test bias or, realized volatility being a bad proxy for future volatility.
Finally, we analyze tail measures such as VaR and CVaR in the charts above. Both distributions center around zero and show negligible estimation error. This suggests that investors can use backtests to assess embedded tail risk, providing a fairly accurate picture.
Conclusion
Quantitative Investment Strategies (QIS) offer immense potential for constructing profitable and risk-controlled portfolios, but they come with inherent challenges, particularly when evaluating backtests. Our analysis highlights that while backtests systematically overstate expected returns—by an average of 4.1 percentage points—they can still provide valuable insights into the risk characteristics of a strategy.
Investors should approach QIS backtests with caution, recognizing their limitations as predictors of future performance. The consistent overstatement of returns across banks, asset classes, and strategies underscores the importance of applying appropriate skepticism and adjusting expectations. However, backtests can be a reliable tool for assessing higher moments, such as volatility and tail risks (VaR and CVaR), which tend to show minimal bias.
In practice, investors should leverage backtests as a complementary resource, focusing on understanding risks rather than expecting precise profitability predictions. By doing so, they can better navigate the complexities of the QIS market and make informed decisions when incorporating new strategies into their portfolios.