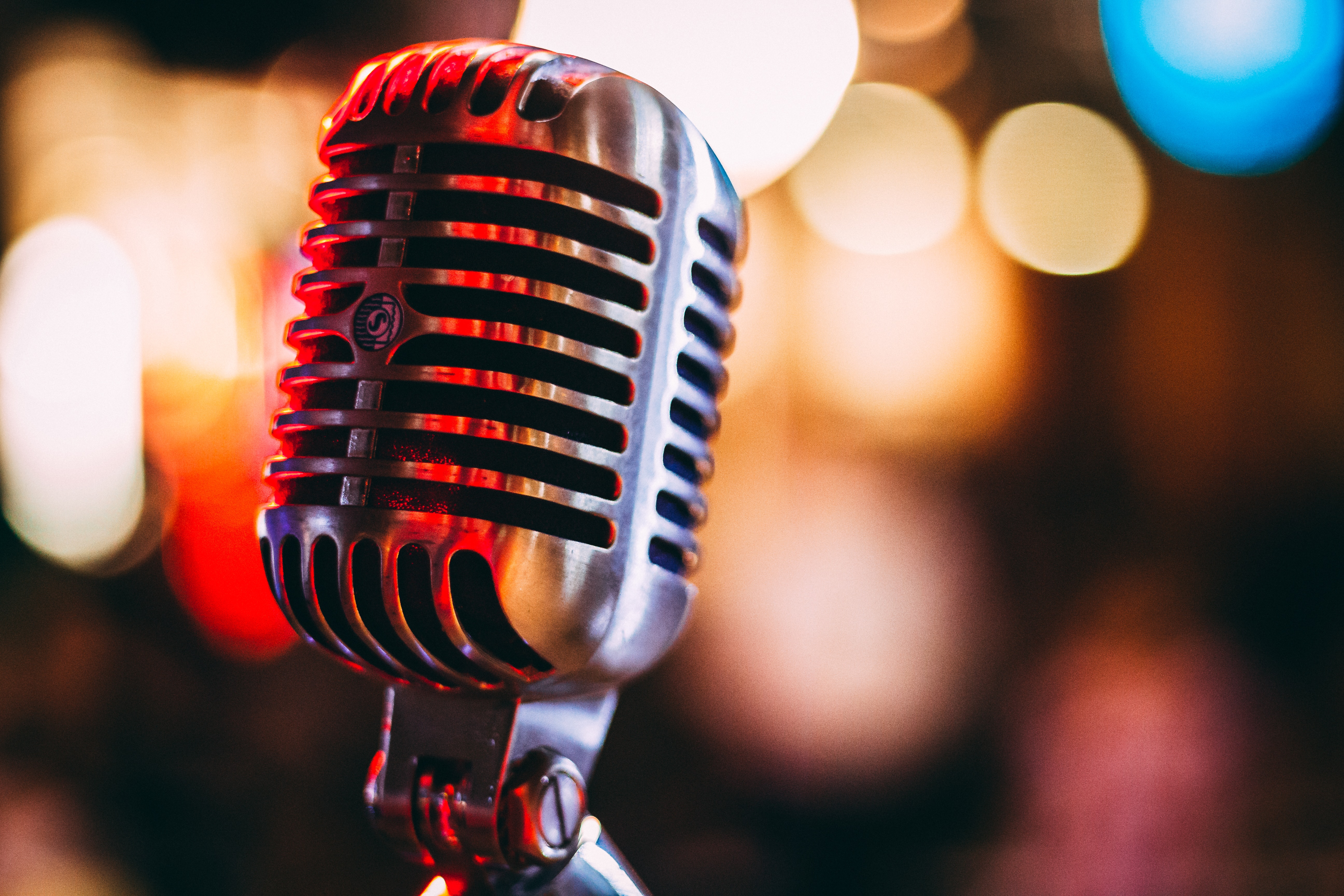
Who Is the Next Star? How Past Performance Can Forecast Expected Hedge Fund Returns
Is past performance indicative of future hedge fund returns? Our analysis delves into patterns and characteristics to provide clarity.
10 min read | Oct 10, 2023
Every investor has come across the typical disclaimer: "past performance is not necessarily indicative of future results". While this might be true for a single investment, given the many qualitative and quantitative aspects to consider when forming expectations about its future performance, one might wonder: does past performance hold any information when analyzing a broader group of investments? If the answer is no, then an investor would be wise to simply ignore past performance when evaluating a new fund. However, if the answer is yes, it's crucial to understand what insights past performance can offer and if there are distinctions to be made among different groups of funds
In this blog post, we demonstrate how Resonanz Capital's quantitative performance rating can be utilized to form better expectations about future returns. The framework is based on past returns, but it doesn't analyze them in isolation. Instead, it compares them to their peers. This is done by utilizing the information ratio relative to a fund's peer group, which places the fund's underperformance or outperformance in relation to the additional risk of that fund. In this post, we'll first show you how this translates to a broad set of different hedge funds and aim to answer the following questions: Which funds are among the best? Are there characteristics that make identification easy, even if you don't have access to RC’s rating?
After gaining a better understanding of which funds are top-tier compared to their peers, we'll focus on whether this rating can assist us in forming expectations about future fund performance. Specifically, we'll examine the following questions: Who are the top performers? Is there a difference in forecast horizon?
Before delving deeper into these questions, we'll outline our dataset of hedge funds and the rating approach in the next section.
Hedge Fund Data & Rating Approach
For our analysis, we use Resonanz Capital’s semi-proprietary hedge fund database, which consists of more than 4,500 unique hedge fund strategies. We typically group these strategies into three buckets: Convergence, Divergence, and Long-Biased. Convergence strategies encompass all Relative Value strategies that operate with a market-neutral profile. Divergence strategies resemble Tactical Trading strategies such as Global Macro or CTAs, which also maintain a market-neutral profile over a long observation period but can take significant direction over shorter time spans. Finally, Long-Biased strategies are akin to fundamental strategies such as Event Driven or Equity Hedge, which usually take a more pronounced market exposure over extended periods.
Using the entire set of hedge funds in Resonanz Capital's database, we rate each fund every month for the past 15 years. In doing so, we primarily utilize Resonanz Capital's quantitative performance rating approach. This method compares a fund's risk-adjusted performance to its peer group in relation to its risk. On a scale of 1 to 5, a higher rating indicates a more attractive risk-return profile of the fund compared to its peer group over the past 5 years. While we typically use a fund's individual peer group for this rating, in this study, we compare a fund's performance to the entire bucket of comparable hedge fund strategies for ease of readability. Using a fund's more granular peer group should yield even more precise results, meaning the outcomes presented in this study should be viewed as a lower bound
Previous Top Performers
Let's now take a closer look at the characteristics of the funds scoring at the very top or bottom of the scale. This will ultimately inform you about the characteristics to look for, even when you don't have access to the performance rating. To do this, we form an equally weighted composite for each month and strategy bucket based on the performance rating. For instance, if a Convergence fund was rated 4 in a certain month, we assign that fund to a portfolio of Convergence funds which all have a rating of 4 and calculate the equally weighted (meaning fund-weighted) return for that portfolio in that month. We repeat this for every rating and bucket each month over the last 15 years. We then estimate the characteristics of the portfolio returns. It's important to emphasize that this analysis is in-sample. However, this is precisely what we're looking for to determine which return characteristics correlate with a good performance rating. In the next section, we'll focus on out-of-sample prediction.
To gain a better understanding of what the rating favors, let's examine the Sharpe Ratio first, as it's the simplest way to describe a fund's risk-return relationship. The chart below plots the Sharpe Ratio for the different portfolios:
It's evident that a good rating correlates with a high Sharpe Ratio, as the Sharpe Ratio increases monotonically with the rating across all buckets. The intuition behind this finding is straightforward. The Sharpe Ratio relates expected returns to risk. This aligns with our approach in the quantitative performance rating, where we assess a fund's risk-adjusted outperformance relative to its peer group and compare it to the additional risks the fund bears in comparison to the peer group. Therefore, it's logical that a fund with a favorable Sharpe Ratio is likely outperforming its competitors and will receive a high rating, and vice versa.
Next, we'll examine the equity beta of the portfolios as a measure of systematic risk:
We find a significantly decreasing beta in the rating across all strategy buckets. That is, better-rated funds have less systematic equity risk embedded in their strategies. This finding is less intuitive than the previous one. As mentioned earlier, we relate a fund's risk-adjusted outperformance to its additional risk. So, let's assume for a moment that all funds within a strategy bucket have some degree of equity risk. The rating approach will then discount the average contribution from equities since this is a common factor among all funds. As such, funds can only outperform by adding alpha on top of that. Our results suggest that funds with a high equity beta are much less capable of doing so than funds with a low equity beta — a finding we've analyzed in greater detail for the Long-Biased hedge fund universe in this blog post.
Lastly, let's examine absolute returns. The chart below displays the annualized returns for the individual portfolios:
For all buckets, we find that the portfolio of funds with the best ratings has significantly outperformed the respective portfolio of funds with the lowest ratings. There's an almost monotonically increasing pattern, with the only exception being Convergence, where the best-rated funds performed slightly lower than the second-best rated funds. While it seems plausible that better-rated funds have delivered superior returns compared to lower-rated funds, it's important to emphasize that this isn't necessarily endogenous to the rating approach. Given that the rating is based on a ratio between returns and risk, a fund with slightly lower returns but significantly less risk than another could be rated higher. However, we find that funds which have offered an attractive risk-return profile have also outperformed their peers on an absolute basis.
All in all, we can conclude that funds with low equity beta, high absolute returns, and a high Sharpe Ratio are most likely scoring high in Resonanz Capital's quantitative performance rating. Naturally, the question arises: what does this tell us about the future returns of these funds? We'll tackle this question next.
Future Top Performers
To estimate the out-of-sample predictability of our quantitative performance rating, we follow a similar approach as before. Every month, over the past 15 years, we construct equally weighted portfolios based on the rating. Unlike before, we don't adhere to an in-sample approach but estimate the annualized return for holding that portfolio for either three or five years. We stick to longer prediction windows to account for the fact that hedge fund investors typically have a longer investment horizon and don't turn over their book of hedge funds rapidly.
The chart below shows the average out-of-sample annualized return for each portfolio over a 3-year holding period:
We observe a clear pattern: out-of-sample returns increase almost monotonically with the rating. This pattern is more pronounced for Long-Biased funds than for Convergence and Divergence funds. This is a very remarkable finding as it suggests that investors can indeed infer something about future returns from past performance, provided they compare it to a relevant universe. For investors to trust such metrics, it's crucial that they offer reliable indications across various investment horizons. We'll therefore focus on a 5-year holding period next. This will also enable us to draw some additional conclusions.
The chart below displays the average out-of-sample annualized return for each portfolio over a 5-year holding period:
Two major observations can be drawn from the chart above. First, we find that annualized out-of-sample returns increase more significantly for Convergence funds than in the previous chart. While the spread between the portfolio with the lowest rating and the highest rating was only 2.9% for the 3-year prediction horizon, it expands to 4.1% for a 5-year prediction horizon. This suggests that our quantitative rating approach can identify Convergence funds that consistently outperform their peers over an extended timeframe. However, over shorter periods, there might be instances of slight underperformance, which are then counterbalanced by stronger outperformance later on as we can directly conclude from the lower 3-year spread.
Second, we observe the exact opposite for Long-Biased funds. While the out-of-sample spread between the best-rated funds and the lowest-rated funds was 7.8% for the 3-year prediction horizon, it narrows to 1.9% for a 5-year prediction period. This indicates a short-term performance trend in fundamental hedge fund strategies. However, these strategies seem to have a limited capacity to generate systematic alpha against their peers. Consequently, it becomes challenging to pinpoint expected outperformers over a longer horizon
Conclusion
In this post, we demonstrate how Resonanz Capital's quantitative performance rating approach can be employed to form expectations about future fund performance. We highlight that funds with high absolute returns, low equity beta, and a high Sharpe Ratio are among those that have outperformed their peers.
Using the rating approach to predict future returns, we discover that the top Convergence and Divergence funds consistently delivered alpha against their peers. This enables investors to form expectations about future returns over a longer time horizon. Over shorter time frames, predictions are slightly less accurate due to additional factors to consider, such as short-term trends and mean reversion, which could result in temporary underperformance for these funds. In stark contrast, we observe the exact opposite for more fundamental, Long-Biased strategies. Based on our rating, we can identify trends in Long-Biased funds over shorter durations. However, the predictability wanes for extended investment horizons, suggesting that we cannot pinpoint systematic alpha against peers. In essence, this indicates that stars rise and fall more frequently for Long-Biased managers compared to other strategies. This also implies that it's more challenging for investors to identify top-tier Long-Biased funds based on past performance than it is for other strategies.
As always, please feel free to reach out if you have any questions.