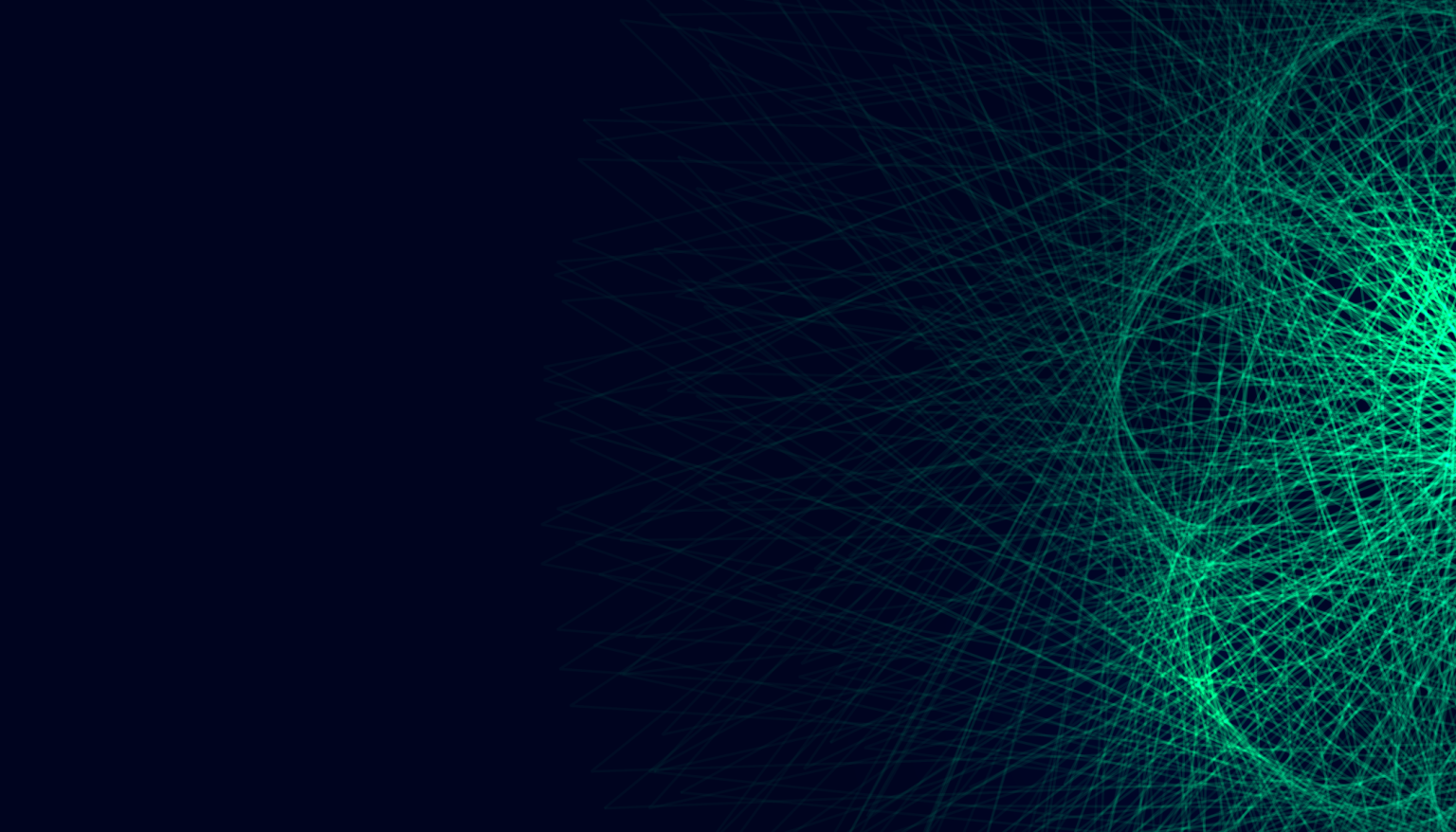
The RC Hedge Fund Crowdedness Index
Examining crowdedness in factor investing, linking high exposure to RC factors with elevated risk and market volatility.
7 min read | Jun 13, 2023
This is the second part of a two-part publication regarding crowd sentiment in factor investing. In the first part of this analysis, Hedge Fund Crowdedness – Resonanz Capital we discussed what crowd sentiment is and why investors should closely monitor it. This version includes the empirical evaluation of crowd sentiment in the equity hedge and event-driven hedge funds.
The present Part II includes visualizations and a brief discussion of the findings for equity hedge and event-driven strategies. We provide insights into which factors investors should pay attention to and stress the leading-edge research that Resonanz Capital (“RC”) applies to monitor risk using Quantitative Investment Strategies (QIS). We group these factors as RC factors because they gauge different risk premia across several asset classes and styles. QIS are provided by investment banks and brokerage houses. Crowdedness index allows investors and investment managers to actively monitor bulk beta exposure and apply factors for both risk and portfolio management.
Data
The analysis includes monthly returns from January 2005 to April 2023.The dataset consists of monthly fund returns across equity hedge, event-driven fund strategies and styles sourced from RC’s proprietary database, which includes more than 4000 individual hedge funds. Before applying the quantitative factor analysis, it is reasonable to question how the return factors relate to each other. The answer provides an indication of the data-dependent clustering.
The method identifies two clusters of factors: a cluster comprising carry/beta, and momentum/low-volatility/liquidity cluster. Exhibit 1 shows the heatmap of the factors considered in the analysis of the crowdedness for the period from January 2005 to April 2023. Obviously, we cannot distinguish the factors on their type of asset class property. The factors are separated based on return and style properties.
Exhibit 1: Heatmap of Factor Correlations
Whereas the funds available for the analysis in the database were slightly more than 400, at the beginning of 2023, their total number increased with time significantly. The sample comprises single hedge funds from various styles, including equity hedge, event-driven, CTA, credit long/short, relative value, global macro, convertible arbitrage, distressed debt, and others. The larger the number of funds, the more reliable and both statistically significant and economically meaningful the estimation of crowdedness becomes. The analysis of crowdedness begins in 2005, which coincides with a period of heightened interest in hedge funds and factor-based quantitative strategies.
Crowdedness Index and Risk
The approach to measuring crowdedness has been extensively described in Part I. The analysis of fund crowdedness reveals that from 2008 to 2011, there was a gradual increase in crowdedness, reaching its highest-level exceeding 30%. This indicates that hedge funds consistently increased their exposure to the RC factors during this period. However, after reaching the peak, crowdedness sharply declined to 5%. Subsequently, the fund exposure rapidly increased, reaching the maximum level again exceeding 30%. The peak in crowdedness can be readily attributed to the heightened factor exposure during the outbreak of the Covid-19 pandemic. The results indicate that the 30% threshold represents a significant point of crowdedness in hedge funds. Once this threshold is reached, crowdedness tends to decrease again. The current level of crowdedness suggests that the factor exposure of the funds is elevated.
Exhibit 3: Overall Crowdedness and the Cboe Volatility Index
Source: Resonanz Capital, Bloomberg, LLC.
Exhibit 3 shows the crowdedness and compares it to the general metrics used to measure crowd sentiment and fear in the markets – the Cboe Volatility Index (VIX Index). The volatility behavior exhibits three regimes – low, medium, and heightened volatility. The regime of middle-level volatility is the most prevalent in the market, as observed prior to the Covid-19 pandemic and the war in Ukraine. During times of volatility spikes, the crowdedness of the hedge funds increased. Since 2020, the pickup in crowdedness is associated with a cluster of medium volatility. In other words, the crowdedness remained high in line with the higher levels of equity market volatility. If the Cboe Volatility Index is considered a risk indicator and it rises in times of higher risk, and the cumulative crowdedness measures the number of funds with exposure to factors, then the increase in risk is closely associated with higher levels of systematic exposure to the RC risk factors.
Monitoring and Analyzing Crowdedness
Investing in factors premia gauged by the different QIS we use in our analysis typically carries the risk of a factor strategy becoming overcrowded, which should be avoided and monitored. We have already discussed the quantitative investment strategies and risk premia in our previous publications – Hedge Fund Returns and Alternative Risk Premium Factors. The reason is that once a bulk of hedge funds try to unwind their exposure to a factor, the factor returns plunged as a consequence of the massive sell off. The term often used is the “stupidity of crowds”. This is why it’s important to estimate both a single-factor-specific crowdedness measure and an overall factor crowdedness that explains the factor exposure within a specific cluster. Since factor exposure and factor premiums are time-varying, rolling window estimation is necessary to detect crowdedness exposure that is not detectable using traditional fixed-period time frames.
By examining the crowdedness in detail, investors can not only monitor their current fund exposure but also investigate the drivers of crowdedness, which may differ slightly from the sum of all factor exposure. Specifically, even if the cumulative crowdedness is the sum of the positive less negative factor exposure across all factor crowdedness metrics, the cumulative factor crowdedness might be driven by some specific factors. Considering the cumulative crowdedness as a risk indicator is essential for hedge fund investors. Therefore, running a LASSO model on the changes in crowdedness provides insights into which factors become relevant, particularly when monitoring overall factor risk.
Using the LASSO approach, the results for the cluster of funds identify the relevant factors. Note that the LASSO model shrinks coefficients that are not significant to zero.
We track the cumulative crowdedness estimations using the RC factor set. The purpose is to investigate the possible drivers of the crowdedness index, that 1) might made the crowdedness replicable, and 2) to identify which, if any factor should be closely monitored. If there is a factor that specifically drives the cumulative crowdedness, then this factor should be considered as a major risk driver for factor investing.
Exhibit 5: LASSO Model on the Change of the Crowdedness Index
Source: Resonanz Capital
The LASSO coefficient plots in Exhibit 5 show that only three factors plus an intercept of -0.012. Moreover, a change of the RC Commodities Liquidity is associated with 16.5% change in crowdedness index (coefficient is -0.16). In addition, the beta of the RC Equities Size factor is -0.10, and the beta of the RC Rates EUR Beta factor is -0.03. However, no other factors explain the change of crowdedness behavior. This suggest that the crowdedness index we propose is more than the sum of the partial exposure of the hedge funds.
Conclusion
The overall conclusion is that the RC factors are currently crowded, but below the threshold level of 30%. The RC factor crowdedness increased since the Covid-19 pandemic and the war in Ukraine. Increased levels of crowdedness are observed in periods of heighten volatility and are generally less desired as investors try to exit common strategies – a phenomenon known as herding. Therefore, not only less crowded factors and strategies should be preferred, but portfolios constructed with less crowded factors are also robust against market corrections and investor sentiment.