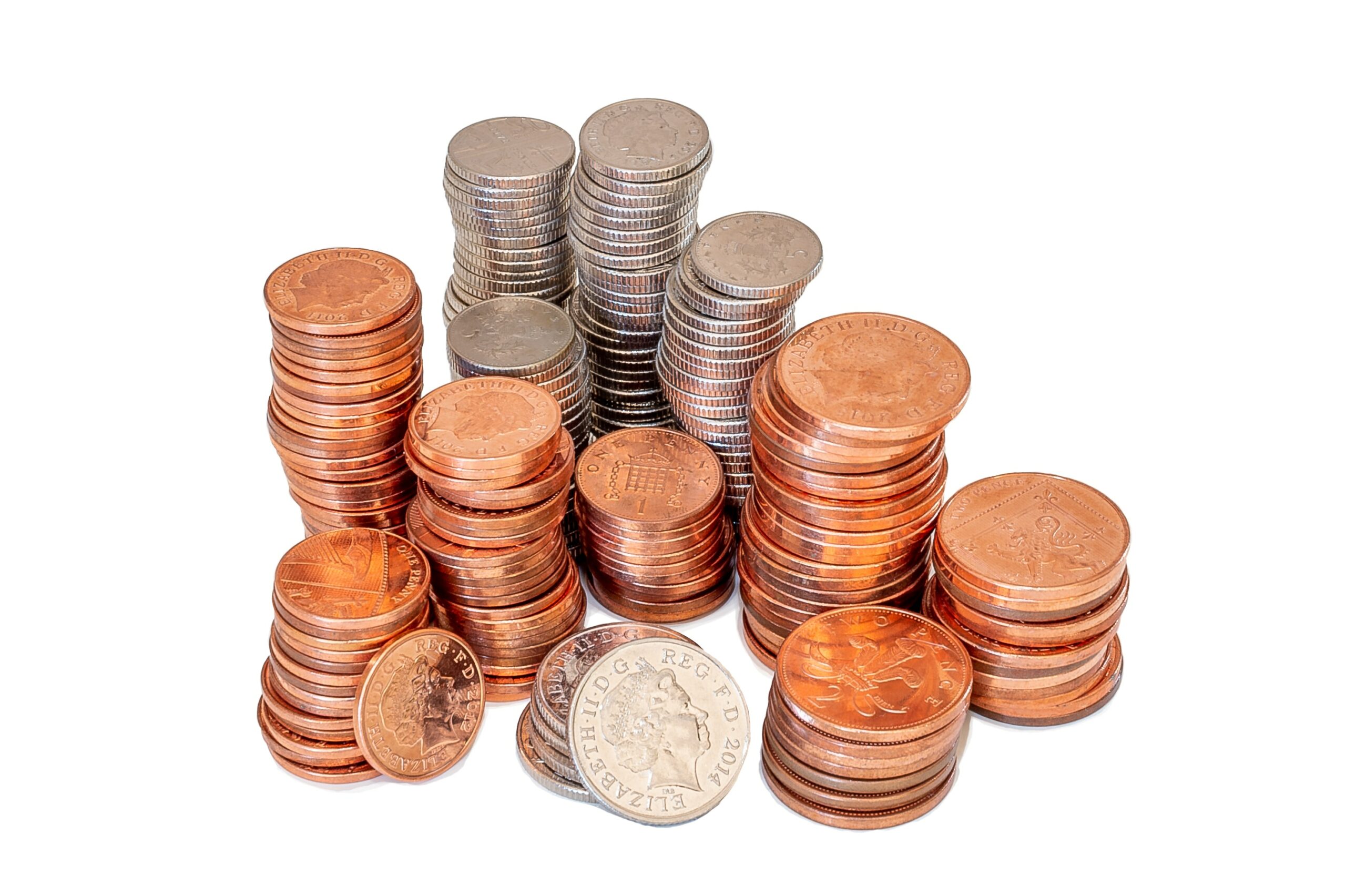
The Myth of Talent in Multi-PM Platforms: Unraveling the True Drivers of Alpha Generation
Multi-PM platforms' high returns come at a cost. Talent has marginal impact on alpha. Risk management and diversification are key factors in success.
7 min read | May 2, 2023
Over the past few years, Multi-PM platforms have gained significant traction due to their ability to deliver high returns, low volatility, and minimal correlation to equity markets (refer to The Exclusive Club of Multi-PM Hedge Funds). Despite their impressive risk-adjusted performance, these platforms tend to rank among the priciest funds in the industry (see The Cost of Alpha Generation) and are gradually deteriorating their liquidity terms (see Are Top Hedge Funds too Hot to Invest). Multi-PM platforms often justify these trends by emphasizing the need to attract top talent to maintain their high-caliber risk-adjusted returns. This raises the question: should investors willingly accept potentially illiquid and costly funds in exchange for entrusting their wealth to the most skilled portfolio managers (PM)?
In this article, we critically examine the argument put forth by Multi-PM platforms, breaking down the components of a Multi-PM’s value generation process. We demonstrate that, contrary to popular belief, talent has only a marginal impact on alpha generation.
The Multi-PM Platforms at a Glance
At its core, a Multi-PM platform’s business model involves employing multiple portfolio managers (PMs), each overseeing their own book and team on behalf of the fund. This arrangement often appears mutually beneficial for both PMs and the platform. Individual PMs and their teams may prefer joining such a platform rather than launching a standalone fund, as it enables rapid scaling of the PM’s trading book, provides a cutting-edge trading setup, and leverages the platform’s market power for improved spreads with prime brokers. For the platform, employing numerous PMs is advantageous, as it facilitates the creation of a well-diversified, multi-strategy portfolio comprising experts in individual trading strategies, with relatively high overall capacity (see Multi-PM Platforms How Much Can a Person Manage).
However, Multi-PM platforms incorporate more elements than merely hiring top-tier PMs. For instance, to mitigate downside risk and prevent cross-contamination between trading books, these platforms have developed some of the industry’s most sophisticated risk management systems. One key aspect of this evolution is the establishment of stringent, individual risk limits for every PM. Additionally, Multi-PM platform books offer extensive diversification across asset classes, strategies, and individual risk-takers. These platforms also possess the agility to swiftly scale PMs’ books up or down in response to sudden changes in investment opportunities. Considering the multitude of factors in a platform’s investment process, it is difficult to pinpoint the sole driver of alpha generation through qualitative assessment alone. In this study, we simulate a typical platform to examine the various effects of the investment process individually.
The Multi-PM Model
We simulate a model Multi-PM platform to isolate and evaluate individual components affecting value generation. We specifically focus on the impact of PM quality. Utilizing a semi-proprietary database of over 10,000 hedge funds as a proxy for individual PMs, we assume the ability to invest and liquidate at any time. Consequently, our results are purely analytical, as real-life scenarios would involve capacity constraints and varying liquidity terms.
Each month, we categorize the hedge funds into three groups: Convergence, Divergence, and Long Biased. We rebalance our Multi-PM portfolio monthly, taking all already invested funds of a bucket and add the best funds in terms of 18-month rolling Sharpe Ratio to this set such that the total number per bucket equals 35. Using the invested and best uninvested funds in each category, we conduct a portfolio optimization with a rebalancing penalty to determine the monthly portfolio weights.
We assume each PM has a drawdown limit of a -1 sigma move on annualized volatility. If this limit is reached at month-end, the book is unwound at net asset value (NAV), and the PM is blacklisted from future investments.
We run the simulation for every month between January 2012 and December 2022. The chart below displays the capital allocation to each bucket over time:
Apart of a certain degree of fluctuation, we see that the capital allocation to the styles has been fairly stable in the past, with Convergence taking the slightly largest allocation, closely followed by Long Biased, and then Divergence. The chart below shows the cumulative return of our Multi-PM platform, as well as the average hedge fund return.
On average, our simulated Multi-PM platform invests in 97 different PMs each month, with an average annual PM turnover of 24%. This platform significantly outperforms the average hedge fund investment and equity markets in terms of risk-adjusted performance, generating an annualized return of 6.5% with a volatility of only 2.26%, resulting in an impressive Sharpe Ratio of 2.3. Our model omits the leverage effects typically applied by real-world Multi-PM platforms, making the Sharpe Ratio an ideal performance indicator. In fact, top-tier Multi-PM platforms like Millennium and Citadel posted Sharpe Ratios of approximately 2.5 during the same sample period, suggesting our model accurately represents real-world platforms.
Questioning Talent’s Role in Alpha Generation
To assess the importance of talent in consistently generating alpha, we rerun our model, progressively excluding the top-performing PMs from each style bucket every month. We exclude PMs in 5% increments until we have eliminated the top half of all hedge funds at every month. The bar chart below displays the resulting Sharpe Ratios for various simulation runs, illustrating the impact of talent on performance:
Several crucial observations can be made from the chart above. First, in all cases, our Multi-PM platform outperforms the average, equally weighted hedge fund universe (indicated by the orange line). This outperformance is substantial; while the average hedge fund delivered a Sharpe Ratio of only 0.62, our simulations range between 2.4 and 1.9. Second, we note that annual PM turnover (represented by the green line) tends to decrease as the quality of PMs declines, although it fluctuates. This finding may seem surprising, but it could be attributed to either lower-quality PMs’ returns being more normally distributed with less skewness compared to top-tier PMs, or the fact that mediocre PMs exhibit less dispersion in their risk-return profiles amongst each other, making them less likely to be replaced by new, up-and-coming PMs.
Our most significant finding, however, is highlighted by the blue bars. Overall, we observe only a marginal effect when excluding top-tier PMs from investments. The Sharpe Ratio, initially at 2.3 when including the best PMs, fluctuates and only begins to decline when we exclude the top 35% of PMs. This suggests that there would be no significant negative impact on alpha as long as platforms can source PMs within the top third of all PMs, a narrative quite different from attracting the industry’s best talent. Remarkably, even when considering only the bottom half of all PMs, our model still delivers a Sharpe Ratio of 1.9—more than triple the average hedge fund’s Sharpe Ratio—resulting still in a reasonably good outcome for a platform.
Focus on Other Value-Driving Factors
The value proposition of Multi-PM platforms is multifaceted. However, our analysis shows that talent plays a less significant role in the Multi-PM investment process than often assumed. Excluding top-tier PMs from investments has only a marginal impact on the risk-return profile of Multi-PMs. As a result, the commonly cited rationale by platforms that less liquid fund terms are essential for delivering alpha since they allow to attract talent appears to be flawed. Despite this, our study supports prior findings that Multi-PM platforms have been among the most profitable hedge funds historically. Further research is required to pinpoint the specific aspects of the investment process that drive alpha generation for Multi-PMs. As talent seems less likely, potential drivers could include nimble capital allocation, extensive diversification effects in portfolio implementation, or stringent risk management implemented by the platforms.