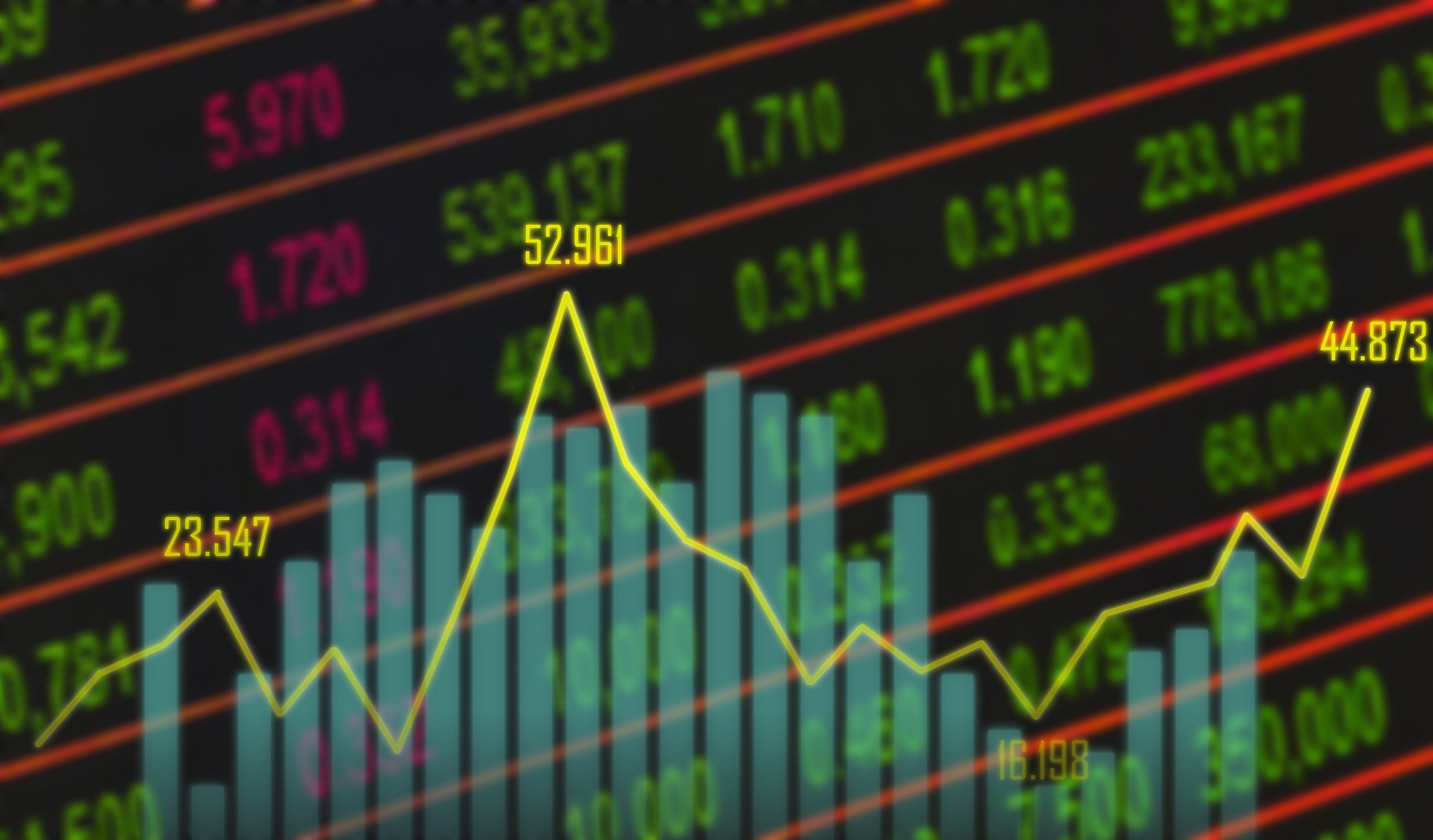
Industry Spotlight: RAM Active Investments
The Head of Systematic Equities from a research-driven investment manager discusses the notable characteristics and evolutions within systematic trading and the strengths and weaknesses of AI in the investment process.
9 min read | Dec 8, 2023
RAM Active Investments' Partner and Chief Investment Officer, Emmanuel Hauptmann, shares insights on the impact of Deep Learning Infrastructure, Natural-Language-Processing and AI on systematic trading strategies.
What are the salient characteristics of systematic trading and how have they changed with time?
Systematic trading relies on a trifecta: extraction of information from data, models to generate trade signals based on this information, and optimal execution.
In recent time data has grown exponentially, the more recent development being the large growth of unstructured text data (news-flow, social media,…) providing large amounts of new information to systematic processes.
Models are also living through some sort of revolution, machine learning providing novel opportunities to better leverage new and larger amounts of data inputs, slowly replacing many more standardized econometric models.
Execution has also in recent years seen a clear development towards higher and higher frequencies, in-line with stronger compute availability and ever lower latency systems.
At RAM AI we have diversified frequencies in our market-neutral strategies since 2021. We deployed statistical arbitrage engines exploiting short-term price-based inefficiencies, with typically a daily horizon, which complement our longer-term investment strategies and increase our long-term Sharpe objective.
How does a bottom-up investment process blend with systematic trading?
To identify attractive long and short stock opportunities, there is a myriad of elements to take into account.
Stocks are impacted by the fundamental dynamics of the company, shifts in sentiment by analysts and market flows from mutual funds or short sellers. In our experience as bottom-up investors, there are hundreds of inputs relevant to an informed investment decision.
Our bottom-up investment process first relies on decision-tree based Value, Momentum and Quality Long and Short stock strategies.
We then built a systematic trading process that combines the hundreds of alpha inputs we have to optimally trade on stocks from our Long and Short strategies. Our objective is to identify the highest potential return stocks from these strategies and have the optimal timing of entry and exit into these long and short opportunities. We are dynamically trading on these strategies’ selections, constantly aiming to maximize the potential return of our long-short book, while maintaining some net Value, Quality and Momentum biases which we are glad to have as investors. A stock needs to go through both the strategy selection filter and the systematic trade optimization layer to make it in the final portfolio.
What is Deep Learning infrastructure and how does it assist the investment process?
Our Deep Learning infrastructure consists in processes we have built to optimally train, validate and test large Artificial Neural Networks (ANNs) models to extract information from data and predict returns.
The first use of our infrastructure is to extract relevant information from unstructured text data, coming from the large amount of news-flow on companies or earnings announcement transcripts for instance. Deep Learning models have become unavoidable to optimally represent text and extract information from it.
The second use of our infrastructure is to build strong models to predict the potential returns of stocks across our strategies. Thanks to our infrastructure we industrialize the hyper-parametrization, training, validation and test of Deep Learning models predicting returns. We then use these return predictions to optimally scale trades on the stocks coming from our Long and Short stock selection strategies.
What is Natural-Language-Processing infrastructure and how has it been impacted by AI evolutions?
Natural-Language Processing (NLP) represents all the models and techniques to systematically treat and extract information from text.
NLP models have strongly evolved in the last years since the advent of the Transformers models which use the Attention mechanism in their architecture (cf. Attention is all you need). Since the first open-sourced model, BERT, five years ago, Transformers have scaled fantastically well (from hundreds of millions of parameters to hundreds of billions of parameters). Large-Language Models now provide us with better representation of text and many new use cases (eg. ChatGPT).
Recently there has been an incredible pace of new Large-Language Models open-source development. Some of these models despite being very good are small enough to be fine-tuned on our compute infrastructure, which we do to extract better information from news-flow on companies and earnings announcement transcripts.
Does Natural-Language-Processing benefit from native speakers in the prompt language?
Large Language Models now are strong across languages and levels of proficiency. Some LLMs are fine-tuned to a specific language or context, which helps further improve results.
At RAM AI we use Large Language Models to extract information from news on companies. There are dozens of thousands of news on the stocks in our investable universe every day and being able to get the information from these news systematically into our process is a strong value-added. Our investment process can integrate these news sometimes days before analysts revise revenue or earnings estimates on the stocks, so it helps us to integrate much faster the information from new events on the firm (product announcement, guidance, management change,…).
We also use them to extract information from earnings announcement transcripts and from Q&A sessions with analysts. The sentiment of analysts and management during the Q&A often carry more information than the presentation by management which is often very “polished”.
What are the strengths and limitations of AI in the investment process?
The strength of AI is ability to model complex interaction between data inputs at large dimensionality. The way fundamentals, sentiment, flows impact stocks is not linear, and AI captures that very well. AI has also become unavoidable to extract information from unstructured data.
One limitation of AI in the investment process is the amount of noise in financial data. We find that a strong level of pre-processing of the information is needed for the model to capture the predictive power of the information optimally. This is where our domain knowledge in finance is key, as we are feeding inputs into the model that are consistent and we can interpret. It is also key so we can interpret what information the model relies on for its predictions, the biases it takes on valuations, price action, positioning, so we don’t use it as a “black box”.
We still find that combining our bottom-up investment strategies with our AI-driven trade optimization process is providing better results out-of-sample than if we had a pure AI process, which means that there is still complementarity in the information from the strategies we build over the last 15 years and our AI predictions.
How do Artificial Neural Network architectures generate trading signals?
In our process we combine a variety fundamental, sentiment, positioning, momentum inputs with short term price, liquidity, risk, technical inputs (close to 500 alpha inputs in total) for the neural network model to predict the most likely out-performers over the short and medium-term. We use the stocks’ short- and medium-term return predictions by the ANN, the correlation to other positions in the portfolio and our in-house market impact model to scale the Long and Short trades.
We tested other approaches where we trained neural networks to directly predict the optimal scaling of positions in the long and short portfolios to maximize a portfolio-level Sharpe objective. The model learnt optimal trade allocations on the base of historical time-series inputs, learning from patterns across timeseries. This approach is more suite for short-term models.
What is Bayesian optimization and how can it dampen biases taken by data scientists?
One complexity in training ANNs relies in the choices one has to make when designing the model and the optimization. What model architecture to choose? How many layers (depth)? How wide (width)? How fast should the model learn from errors in training (learning rate)? How much control for potential overfitting through regularization (L1, L2 penalization of parameters’ scale in loss), how much through random extinction of neurons (dropout)?...
The choices are plentiful and it is easy to see how a data scientist, by testing too many different options, can take biases on the out-of-sample data she/he uses to test the accuracy of the models.
To remove any risk of these biases, we have “industrialized” the process of selecting hyper-parameters, and we are using a Bayesian optimization process which aims to select an optimal set of hyper-parameters with the least number of trials possible, validating the accuracy of the model along the way. We then have a pure out-of-sample test dataset to ensure the return prediction accuracy is strong in timeframes and universes never seen by the model.
This “industrialized” process enables us to train a large number of distinct models with different return objectives, which helps us build a robust prediction by averaging prediction over an ensemble of models.
What are the best and worst environments for systematic trading strategies?
The best environment for systematic trading strategies is an environment of high dispersion of returns, which offers opportunities to play convergence trades on short-term via statistical arbitrage and long-term value biased strategies.
Some of the worst environment come when you have a new type of exogeneous market shock like COVID in 2020, leading to large market moves not easily predictable from history, leading to fast deleveraging across systematic players.
What are the opportunities and challenges with systematic trading strategies going forward?
The opportunities come from the fast growth of data available and the rapid progress of technology, including AI, which should help shape better investment strategies and could provide a relative edge for systematic approaches relative to discretionary ones through time.
The main challenge is the risk that a small number of players build up data and compute capacity so large they prevent most other players from capturing inefficiencies, raising the barriers to entry for smaller alternative players. We have already found for many years that on our lower frequency strategies, inefficiencies and expected strategy returns are in general much higher for instance in the Emerging Markets universe than in the US. It becomes key for smaller systematic players to focus on less crowded market segments where they can have an edge in the implementation of the strategies given their smaller size.