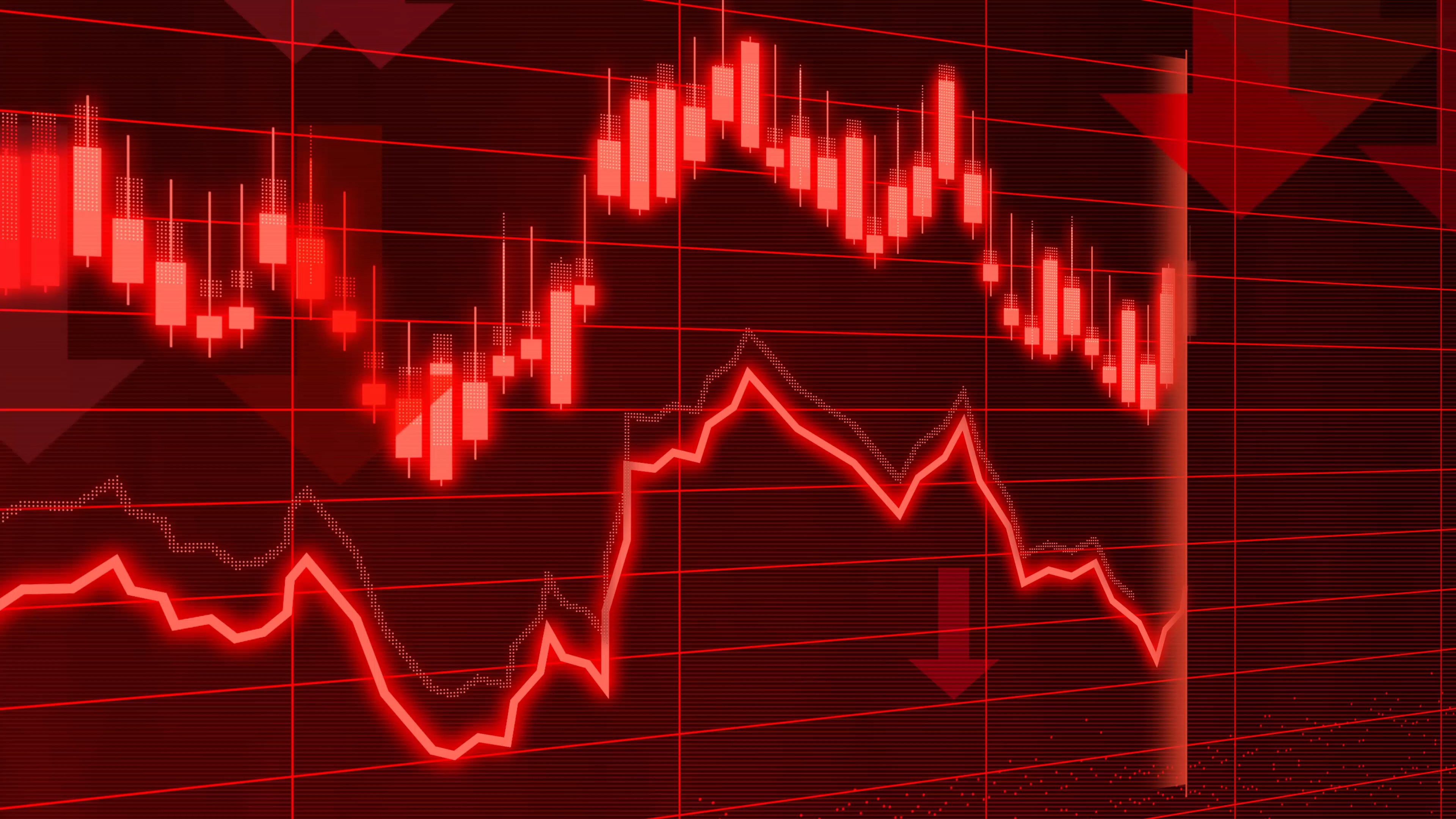
Industry Spotlight: Capital Fund Management (CFM)
Dr. Philip Seager, the Head of Portfolio Management at Capital Fund Management (CFM), shares insights on AI, NLP models and quantitative strategies
7 min read | Sep 6, 2023
Dr. Philip Seager is the Head of Portfolio Management for Capital Fund Management (CFM). We discuss his thoughts on salient characteristics of quantitative strategies
Is Alternative Data the new trend after Big Data?
What is Alternative Data anyway? It used to be anything that was not price, then perhaps anything not on a Bloomberg screen. Big Data implies a data set that is large and unstructured which is increasingly the case for many new data sets we study. I don’t know if Alternative Data is a new trend but it is certainly big business. We now have a large team managing the process from the sourcing and performing due diligence on providers to the treatment, cleaning and testing all the way through to feature engineering before the data set is passed to the research team. The infrastructure we have built to scale up this effort is immense. As much as the barrier to entry to work with large data sets is comparatively lower than previously the barrier to scale up the effort is high and requires significant investment.
How can AI help manage data for the investment process?
Artificial Intelligence is useful for problems with very high signal to noise ratios. In data analysis, then, we can and do use it to complete data sets, filling in holes where they exist, although that data then needs to be used with an appropriate level of care. We also use such automated techniques for detecting anomalies in data and for extracting features in NLP. AI can be used also to fit the solutions of optimization problems which, in certain situations, makes optimization somewhat less heavy computationally.
What is your experience with NLP models and the investment process?
The area of NLP has been a big push for us in recent years. We now have a lot of text data ranging from news articles to earnings transcripts and analyst reports. We have done a lot of work on embeddings and the representation of text, building on the work done in the open-source community. Of course, recent developments, with the advent of ChatGPT, have been exciting in this fast-moving space. These large language models (LLMs) are tremendously powerful in bringing a boost to productivity but we are as yet to successfully use any such LLM technology in our investment process …. but watch this space!
What is the relationship between capacity, trading frequency and crowdedness for quant strategies?
In my experience the higher frequency strategies suffer more from the competition than those on slower timescales as the capacity is naturally limited and thus less able to deliver when joined by the competition. We have developed crowdedness indicators that allow us to detect the competition trading the same signals as us, which is particularly useful, for example, in seeing if an alternative dataset is becoming more widely used. We have been at the leading edge of market impact research for a number of years and that has helped us to understand these effects.
What are the differences between HFT strategies and alpha strategies?
We employ alpha strategies on a range of timescales from systems that trade on an intraday timescale to those that trade on timescales of several months. This spectrum of timescales is very common in quant as opportunities tend to present themselves differently as a function of the timescale one is trading on. High Frequency Trading (HFT) I would say is a business in which one is trying to make money out of the provision of liquidity. It is technology driven, an arms race in reducing latency, and is not an area we are competing in. This HFT space tends to be capacity limited and firms would be trading proprietary capital. We do not engage in proprietary trading and invest in our products alongside our clients.
What are the characteristics of alpha decay?
Alpha decay can occur through crowdedness or through a repeating market pattern disappearing. The faster strategies tend to decay more quickly through crowdedness as faster strategies have less capacity and are more quickly crowded as the competition picks up on the effect. The risk adjusted performance in the absence of costs is also better so it is easier to achieve significance with statistics on alpha decay with these shorter time frames. Patterns that exist due to repeating market behavior may vanish as markets evolve. For example, a pattern that emerges due to a central bank implementing quantitative easing measures will disappear (strategy alpha decay) as the central bank pulls back from such measures.
How has execution efficiency evolved for the strategy?
A very constant feature in markets has been that our cost of trading, which is predominantly due to market impact, reduces as liquidity increases. This seems to be independent of the nature of the liquidity. As more HFT players (and others) have joined the market and liquidity has, in consequence, increased, our slippage per unit of traded volume has significantly reduced. We do, however, invest continually in our execution capability, working on short term predictors, understanding order book dynamics and also measuring and modeling costs. This is the result of many years of research in this space and something we would consider to be an edge relative to our competition. We develop all algorithms and infrastructure in-house and regularly benchmark our execution capabilities against other providers.
How relevant are back tests to the investment process?
We do a lot of backtests! Which is perhaps unsurprising for a quant firm. However, backtests are taken with the pinch of salt they deserve. In-sample biases, or buying positive performing noise is the biggest problem in doing strategy research. This is not unique to quant though, I would argue, with discretionary players investing in patterns they have seen that worked previously. For these reasons backtests are really only used as a guide to future performance. The advice of regulators should be heeded on this point that past performance is not a good indication of future results! Instead, the backtest is a small part of what helps us decide, with an understanding of the driving mechanism behind the strategy also important to add significance to the result.
How do you see quant strategies evolving over the next decade?
I think the proliferation of data will be a bigger and bigger part of the quant space. Where there is data there is information and added value for those able to exploit it. The problem is being able to build the infrastructure and the team that is able to exploit it to the full. To me that is the biggest challenge. Scientific advances have always been made in a collaborative fashion and this is no exception. Being scientists ourselves we are big believers in this ethos and recruiting young, driven researchers and data scientists and scaling up this activity is what has driven our efforts over the past few years. It is our belief that our pipeline and infrastructure puts us in a good position for a future that will favor the bigger established players able to exploit wide and varied, structured and unstructured data sets.