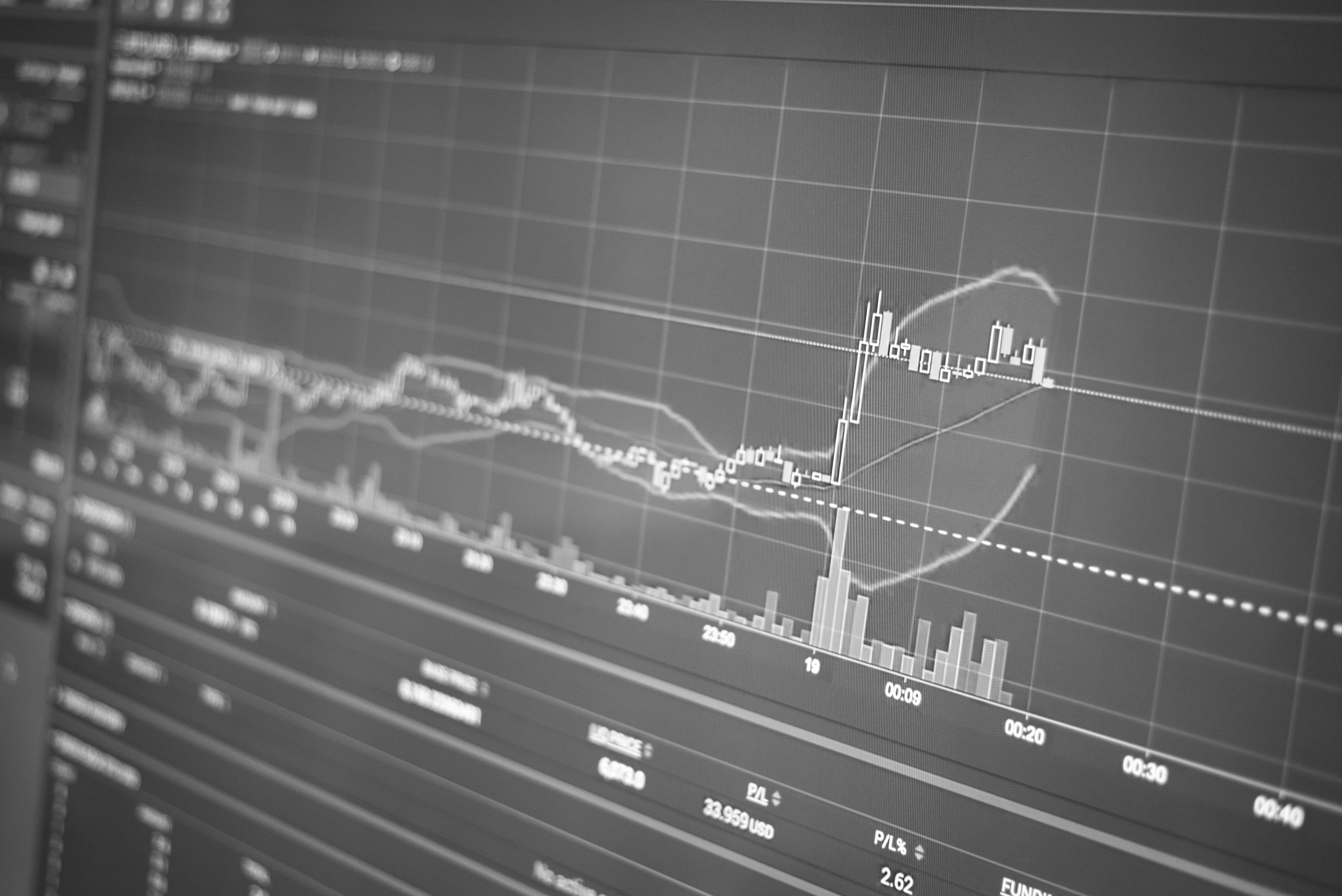
Industry Spotlight: a renowned quant speaks on data science and investing
Dr. Joseph Simonian shares his insights on data analysis, causal networks, and the investment process.
5 min read | Jul 11, 2023
Dr. Joseph Simonian is a data scientist and co-editor of the Journal of Financial Data Science. He shares his thoughts on the impact of data science, causal networks, and reinforcement learning on the investment process.
How is data science revolutionizing investment practice?
I believe that the primary way that data science is revolutionizing investment practice is through shifting the emphasis of statistical models from theory and an emphasis on explaining the past to predicting the future. As we know, machine learning and data science algorithms are often geared towards prediction and/or pattern recognition something that traditional econometric models are not good at because they are fashioned primarily as a means to explain the past and drive economic theory (an orientation promoted by the Cowles Commission in the early 20th century) but are not really equipped to forecast and predict in a reliable manner. So the placing of investment practice on more solid empirical footing is what I see as the primary contribution of data science to investing.
How can AI model complex open ended systems like the weather or investing?
This is a good question. No doubt investing is an extremely complicated endeavor because of its open nature. Nevertheless, because machine learning and data science have pattern recognition among their primary areas of focus, the processing and treatment of complex patterns in time series and other types of data can presumably be assisted by these types of models. In contrast, traditional statistical models do not even have the formal means to address some of the complexities we find in investment data. So while there is no quick fix or magic pill to solve all our investing challenges, I'm confident that data science techniques can be a powerful tool in investors arsenal.
What are the current challenges faced by data science analysis and investment practice?
From my standpoint, there are two primary challenges in applying data science to investing. The first is that more data science frameworks need to be developed that are specific to financial data and investment applications. This is because financial data has some unique characteristics that are a result of its source in human behavior. In fact, all the behavior we observe in the markets, from momentum to volatility clustering, is a result of human action. But this is a work in progress and is moving along at an impressive pace. The second challenge is in the practical application of data science to financial problems. Many applications of data science to investing still seem to be driven by simplistic textbook-type models that don't address the complexities of investing. A further issue is that too few quantitative investors seem to be conversant in both traditional econometrics and data science, and hence are unable to determine which type of tool to use when. This is something that needs to be addressed in the education that future "quants’ receive.
How would you explain causal networks?
Causal networks, also known as Bayesian networks, are graphical representations of cause and effect relationships between variables. The variables are represented by nodes and the relationship between causes and effects is represented by the edges or arrows connecting the nodes. The arrows indicate the direction of causality. Now the structure of a causal network can be based on acquired knowledge about a causal relationship or assumptions about it, and once we posit the structure for a causal relationship, then we can also add probability values to model the strength of these relationships. One of the major ways that causal analysis is conducted is by modeling interventions. In other words, we can look at how the cause and effect relationship between variables changes based on specific changes in the variables. Causal networks can also be used to enhance our predictive capabilities, as they can help us identify the key variables in a model that aid or hinder successful forecasting.
What are the disadvantages of causal analysis and the investment process?
I wouldn't say "disadvantages". I would say "challenges". The challenge to causal analysis in finance is that it is often much more difficult to identify the causal drivers of financial phenomena when compared to natural phenomena. This is because in finance, we don't have the ability to conduct closed experiments in the way that we do in natural science. The inability to conduct closed experiments is also problematic because it makes it harder for us to filter out confounders from our studies. Confounders are variables that influence both the dependent variable and independent variable. Accounting for them is important in causal analysis but particularly difficult in finance.
What is reinforcement learning?
Reinforcement learning is a machine learning framework that models agents decision-making in specific environments. As the agent progresses through different states (configurations of their environment) they take actions and receive rewards and punishments for different decisions. Over time they learn policies or rules that will aid them in further decision making based on the rewards or punishments they have received in specific states. I should mention that reinforcement learning is not a deterministic framework, as agents are allowed to explore and take actions that they have not taken previously in order to see if their novel actions will improve their utility over time.
How can game theory and reinforcement learning enhance risk models?
Well both game theory and reinforcement learning involve the consideration of explicit rewards and punishments as consequences of action. Moreover, they also involve the application of different strategies to specific situations. This is very much akin to risk management, where we have to consider the downside (punishment) as well as the upside (reward) to any decision. What is unique to game theory and reinforcement learning is that risk can be considered from the agent's standpoint. This makes these frameworks complimentary to purely statistical approaches to risk modeling.