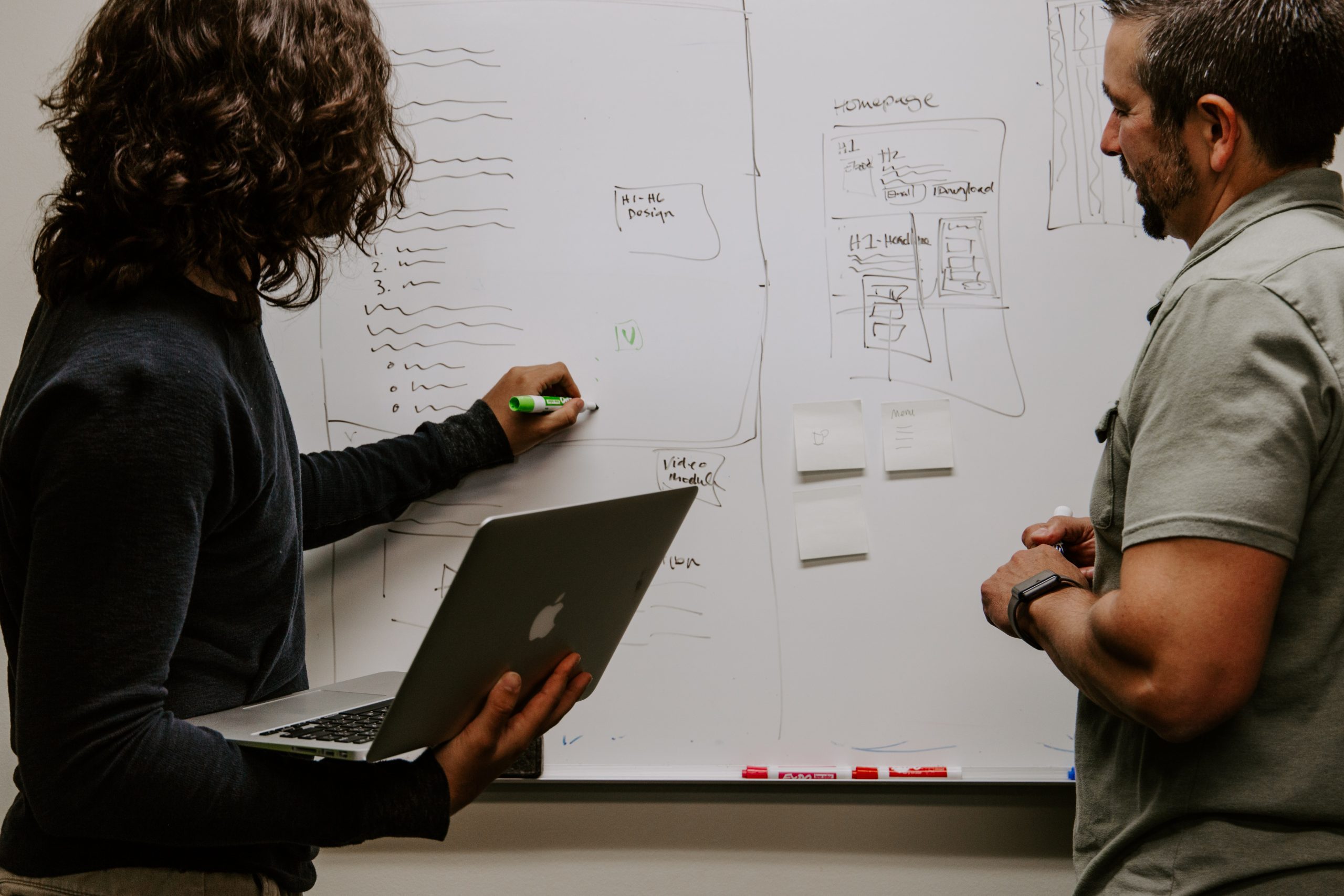
Identifying Alternative Risk Premium Factors
QIS indices provide 46 RC Risk Premium factors, aiding hedge fund analysis and evaluation of modern investment strategies.
5 min read | Mar 15, 2022
Why Do We Need (New) Risk Factors?
In determining a fund’s ability to generate alpha, or in evaluating a fund’s market risk, the sensitivity of the fund to risk factors is usually considered. In 2004, Fung and Hsieh proposed to measure a fund’s risk or alpha using 7 directly tradable risk factors, as these factors could explain a large portion of hedge funds’ monthly return variation.
Fung and Hsieh’s 7-factor model has since become the workhorse for hedge fund analysis in research. However, hedge fund trading strategies have evolved since 2004, and the markets themselves have become more complex. Therefore, to keep up with the markets, one may want to look at a wider variety of indices.
Quantitative Investment Strategy indices (QIS) are products offered by investment banks. They are based on systematic, complex investment strategies across different asset classes and aim to harvest different alternative risk premiums. As such, these indices may be a fruitful tool for assessing a fund’s systematic risk, as these indices are based on systematic trading strategies that can also be found in hedge funds. Therefore, they may be the primary candidate to assess the risk inherent in a hedge fund strategy.
The universe of these indexes, however, is very large and most of these indices are non-public and some offer limited data quality. Therefore, the question arises how to obtain, clean, and aggregate these indices to construct a limited number of risk factors that inherent different information.
Calculating Risk Premium Factors
We collect more than 1000 different QIS indices from all major investment banks. Most of these indices are calculated daily and are all tradable via swaps. To structure our dataset, we apply a combination of qualitative and quantitative grouping techniques:
First, we classify all QIS indices based on the asset class and alternative risk premium they focus on. For asset classes, we distinguish between Commodities, Credit, Equities, FX, Multi-Asset, and Interest Rates, and group the alternative risk premia into Alpha, Beta, Carry, Event, Liquidity, Low Volatility, Mean Reversion, Momentum, Multi-Premia, Size, Seasonality, Value, and Quality.
We then apply quantitative filters to identify all indexes within an asset class and alternative risk premium combination that share similar characteristics and are most common across providers. A volatility-weighted portfolio of all remaining indices then serves as the RC Risk Premium factor for that asset class and alternative risk premium combination. This method gives us 46 different RC Risk Premium factors.
RC Risk Premium Factors
The figure below shows a heat map of the correlation between the different RC factors:
Figure 1: Correlation Heat Map for Alternative Risk Premium Factors. Source: Resonanz Capital
From the figure above, we can see that most of the factors are relatively uncorrelated with each other. In fact, the average pairwise correlation is almost zero (mean: 0.03, median: 0.01). Thus, the RC factors convey orthogonal information that is potentially useful in explaining a variety of different hedge fund strategies. Of course, the pairwise correlation varies, and individual factors may have a correlation significantly below or above zero. We find the lowest overall correlation of -0.46 between the RC Equities Mean Reversion and the RC Multi-Asset Volatility factor. In contrast, the highest pairwise correlation of 0.82 is between the RC Commodities Carry and the RC Commodities Liquidity factor.
The table below shows the median pairwise correlation of the RC factors for the different asset classes:
Asset Class | Number of Factors | Median Correlation |
---|---|---|
Commodities | 8 | 0.02 |
Credit | 4 | 0.12 |
Equities | 12 | 0.03 |
FX | 9 | 0.05 |
Multi-Asset | 6 | 0.12 |
Rates | 7 | 0.14 |
Overall, it can be seen that the Commodities (0.02) and Equities (0.03) factors have the lowest median correlation. On the other side of the spectrum, rates factors (0.14) have the highest median correlation. The table below shows the median correlation between the different risk premium groups:
Risk Premium | Number of Factors | Median Correlation |
---|---|---|
Alpha | 2 | 0.03 |
Carry | 6 | 0.10 |
Event | 1 | nan |
Liquidity | 5 | 0.01 |
Low Volatility | 6 | -0.00 |
Mean Reversion | 2 | -0.01 |
Momentum | 6 | 0.08 |
Multi Premia | 4 | 0.15 |
Size | 1 | nan |
Seasonality | 2 | -0.02 |
Value | 4 | 0.01 |
Volatility | 6 | 0.21 |
Quality | 1 | nan |
Across all asset classes, Low Volatility factors (-0.00) have the lowest median pairwise correlation. In contrast, Volatility factors have the highest median pairwise correlation (0.22). The above tables also show that Equities offer the largest number of different risk premium factors (12) and Credit the least (4). In addition, each asset class has a Carry, Low Volatility, Momentum and Volatility factor (6), while Event, Size and Quality are only represented in one asset class.
What did we learn?
QIS indices could be a useful tool for assessing overall risk in modern hedge fund investment strategies. However, in addition to their potential value added for fund analysis, they also have clear drawbacks: The data is usually non-public, scaled differently, and the number of individual indices is too large to be useful in identifying common risks.
We collect more than 1000 QIS indices from all major providers. We further use a combination of qualitative and quantitative methods to structure the dataset and identify systematic risks. We extract 46 RC Risk Premium factors. These factors inherent largely orthogonal information and are therefore a potentially useful tool for evaluating modern investment strategies.