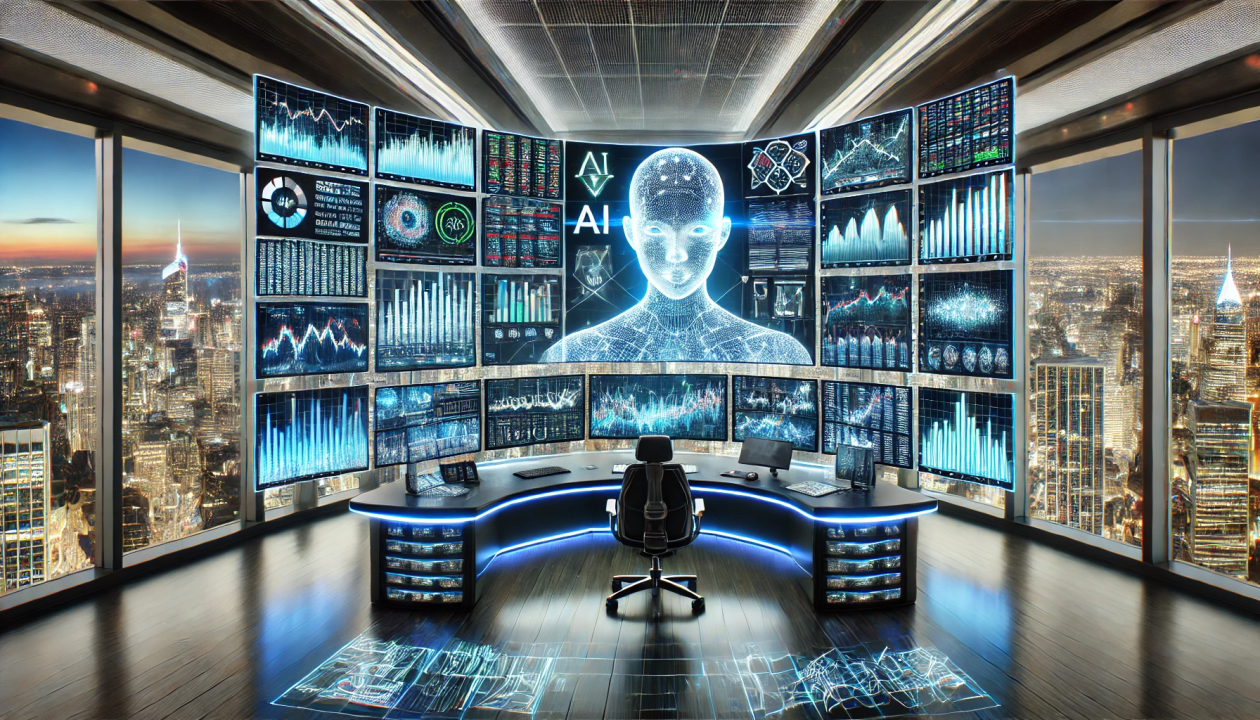
How Hedge Funds Are Really Using Generative AI — And Why It Matters for Manager Selection
How hedge funds are using generative AI to improve research, trading, and efficiency—what allocators need to know for smarter manager selection.
12 min read | Apr 20, 2025
The hype around generative AI (GenAI) in financial services is loud—but for hedge fund allocators and due diligence professionals, the signal is what matters. But for these professionals, it’s critical to understand not just whether a manager talks about AI, but how they are actually using it to sharpen their edge, reduce risk, and operate more efficiently.
Generative AI has swiftly transitioned from a speculative concept to a tangible tool reshaping the financial landscape. While traditional machine learning remains prevalent for tasks like signal generation and risk assessment, Gen AI introduces new possibilities for insight creation, market scenario exploration, and automation within investment processes.
Industry Adoption: Where Hedge Funds Stand Today
Generative AI is no longer in pilot mode across the hedge fund world—it's becoming a core enabler in day-to-day operations. Many managers have already integrated GenAI tools to support functions like internal research, document summarization, and code automation. In some cases, firms are going further, developing proprietary AI platforms tailored to their data environment and compliance needs.
One particularly practical use case gaining traction is retrieval-augmented generation (RAG), which allows investment teams to search and query internal research archives more intuitively—surfacing insights that would otherwise remain buried in PDFs or internal wikis.
On the operational side, firms are building internal governance frameworks to manage the risks associated with GenAI. Chief Technology Officers, Chief Operating Officers, and Chief Compliance Officers are increasingly responsible for shaping AI policies that define what data can be used, how tools are accessed, and where human oversight remains essential.
In short: adoption is real, but maturity varies. The differentiator isn't just who is using AI—but who is using it responsibly, securely, and in a way that aligns with their investment process.
How Hedge Funds Are Using Generative AI Today
While generative AI is often associated with flashy demos and viral headlines, its real impact in hedge funds is measured by its ability to quietly improve workflows and decision-making behind the scenes. For firms willing to move beyond experimentation and integrate GenAI thoughtfully, the technology is already proving useful in several areas of day-to-day operations. The following are some of the more grounded and effective use cases emerging across the industry:
- Enhancing Trading Strategies
Systematic and quant funds are using GenAI to identify subtle market patterns, sometimes with the help of synthetic datasets generated via tools like GANs. These models help stress-test trading strategies against alternative market conditions before deploying real capital. - Improving Market Predictions
Managers are training LLMs on macroeconomic datasets and earnings calls to surface patterns that would be too labor-intensive to catch manually. This supports thematic baskets, trade ideas, or timing overlays. - Optimizing Portfolios
For fundamental managers, GenAI can assist in modeling optimal position sizing, simulating risk scenarios, and backtesting with alternative data inputs. The focus is on augmentation, not automation. - Operational Efficiency
From auto-generating investor letters to summarizing regulatory documents, GenAI reduces time spent on administrative tasks, freeing up investment teams for higher-impact analysis, GenAI tools reduce manual effort and allow hedge funds to get more value out of their existing datasets. Some firms are even generating synthetic data to backtest strategies in low-signal or illiquid markets. - Assisting with Regulatory Compliance
With the ability to monitor vast volumes of transactional and communications data, GenAI can flag anomalies or potential compliance issues early—an increasingly valuable capability in an environment of growing regulatory scrutiny. Some funds use GenAI to review internal communications and flag potential red flags or information-sharing risks. This adds a new layer of operational oversight—especially useful for firms managing scale or complexity
The main advantages hedge funds say will come from embracing Gen AI tools
Source: “Getting in Pole Position: How Hedge Funds Are Leveraging Gen AI to Get Ahead”, AIMA, 2024
Real Benefits Allocators Should Pay Attention To
The adoption of generative AI in hedge funds isn’t just about keeping up with technology trends—it’s about solving real business problems. From improving investment outcomes to reducing operational friction, GenAI is starting to show measurable value where it's been implemented thoughtfully. These benefits are not theoretical; when evaluating hedge fund managers, understanding the outcomes of GenAI use—not just the tools—is key. Below are tangible benefits that may signal stronger operations or potential alpha.
- Improved Decision-Making:
GenAI models help hedge fund analysts process alternative data—like satellite imagery, social media sentiment, or earnings call transcripts—to extract insights that inform trading decisions. For example, a fund might use a large language model to scan and summarize dozens of earnings transcripts overnight, highlighting sentiment shifts or changes in management tone that could indicate forward guidance risk. - Increased Efficiency:
Many firms use GenAI to accelerate mundane but necessary workflows. A global macro manager, for instance, may deploy GenAI tools to auto-generate first drafts of internal market commentaries or investment memos based on structured inputs like GDP releases or FOMC minutes. This drastically reduces the time analysts spend writing, allowing for quicker internal communication and reaction. - Enhanced Risk Management:
Some hedge funds are incorporating GenAI to monitor internal chat logs, emails, and trade activity for compliance red flags or behavioral risk indicators. One fund uses a GenAI tool to review internal Slack channels, flagging language that suggests insider information or unapproved communication around sensitive events—creating a new layer of risk oversight without adding staff. - Optimized Trading Strategies:
Quantitative strategies can benefit from GenAI's capacity to synthesize new signals. One hedge fund is experimenting with generative adversarial networks (GANs) to simulate synthetic financial time series, enabling them to test trading strategies under hypothetical but plausible market conditions—helping validate the robustness of their models. - Cost Reduction:
A mid-sized equity long/short fund replaced outsourced marketing content creation with a GenAI assistant that drafts pitch decks and investor updates, based on portfolio data and house style preferences. What used to take days and multiple revision rounds with an external consultant now takes a few hours internally—cutting costs and turnaround time. - Cost Reduction:
At firms like Man Group and Two Sigma, GenAI is being woven into proprietary research platforms. These tools assist with coding, data discovery, and summarization, giving researchers and PMs faster access to insights and enabling quicker iteration of investment theses. The speed of execution—and ability to bring ideas to market faster—can be a decisive advantage. - Scalability:
As assets under management grow, firms often struggle to maintain research depth across more markets or sectors. GenAI offers a solution. One credit hedge fund used GenAI tools to ingest and summarize thousands of bond prospectuses and indentures, allowing their analysts to cover more securities with greater speed and consistency, even with a lean team. - Fostering Innovation:
By handling routine documentation and first-pass analytics, GenAI frees up time for teams to experiment with new datasets or cross-strategy ideas. For example, a fund exploring ESG integration used GenAI to analyze proxy voting data and sustainability disclosures across dozens of jurisdictions, enabling a faster test of whether ESG screens could be alpha-generating in their specific investment universe.
Uses for Gen AI tools identified by hedge fund managers
Source: “Getting in Pole Position: How Hedge Funds Are Leveraging Gen AI to Get Ahead”, AIMA, 2024
Red Flags and Friction Points
Despite its growing adoption, generative AI isn’t a silver bullet. Hedge funds looking to integrate GenAI meaningfully into their investment or operational workflows need to navigate several non-trivial challenges. These are not just technical constraints—they touch on governance, compliance, and the limits of current machine learning capabilities. Here’s a closer look at the most common friction points and real-world examples of how they’re playing out:
- Data Quality Issues
GenAI models are only as good as the data they’re trained on. If the input data is noisy, incomplete, or biased, the outputs can be misleading. One hedge fund attempting to train a custom LLM on internal analyst notes found that inconsistent formatting and jargon-laden commentary led to unreliable summaries and investment theses that lacked coherence. This highlighted the need to clean and standardize internal content before feeding it into any AI model. - Lack of Transparency ("Black Box" Problem)
Hedge funds must be able to explain how decisions are made—especially when reporting to regulators or investors. GenAI, however, often lacks interpretability. A European long/short equity manager piloting a GenAI-supported stock screener abandoned the project temporarily after failing to explain why the model favored certain low-volume small caps. Without a clear rationale, they couldn’t sign off on trades that couldn’t be justified to compliance or clients. - Reputational Risks
Publicly embracing GenAI without proper controls can backfire. A fund that used an LLM-generated macro commentary in its investor newsletter received pushback when a sharp-eyed LP noticed factual errors in the geopolitical timeline it referenced. Even minor inaccuracies—especially if they appear authoritative—can damage credibility in investor communications or due diligence settings. - Security Threats
Inputting sensitive data into open-access GenAI models like ChatGPT creates potential for IP leakage. One fund's internal trial flagged a developer using real client trade data in prompts to debug a pricing tool. Even though the LLM was technically secure, the practice of entering proprietary data into a public model presented unacceptable security risks. This led to the fast-tracking of a firmwide AI use policy and the development of a private, firewalled GenAI environment. - Over-Reliance on Technology
Some firms risk treating GenAI outputs as objective truth. A macro fund’s analyst team briefly relied on GenAI to summarize central bank statements and interpret policy shifts. But when the AI missed a subtle change in the Bank of Japan's language around yield curve control—something human analysts caught—trust in the tool dropped. GenAI can be a strong support tool, but it still lacks the domain judgment and interpretive nuance of experienced professionals.
Source: EY Parthenon, Oct-Nov 2023
Best Practices You’ll See at the Most Sophisticated Managers
Leading hedge funds integrating GenAI aren’t just buying tools – they’re building thoughtful systems. While the potential is substantial, capturing real value from GenAI requires far more than just plugging into an API. The following best practices—many drawn from early adopters in the industry—offer a practical roadmap for hedge funds to deploy GenAI in a way that’s scalable, compliant, and strategically aligned.
- Invest in Robust Data Infrastructure
The foundation of any successful GenAI strategy is high-quality, well-organized data. One multi-strategy fund began their AI journey by centralizing internal research, market data feeds, and trade logs into a single searchable knowledge base. This not only improved the performance of their GenAI tools, but also unlocked additional value through better internal collaboration. Clean, tagged, and structured data is what turns GenAI from a novelty into a reliable teammate. - Assemble a Skilled AI Team
Rather than hiring a generic “AI expert,” some funds are creating multidisciplinary teams that include data scientists, quants, compliance professionals, and PMs. For example, a New York-based hedge fund hired a product manager with experience deploying GenAI in enterprise settings to bridge the gap between technical teams and front-office users. This approach ensured that the tools being built solved the right problems—and didn’t sit unused. - Evaluate Technology Options Thoughtfully (Build vs. Buy)
Not every hedge fund needs to build their own language model. A credit-focused manager found that off-the-shelf GenAI tools like Microsoft Co-Pilot covered 80% of their use cases—such as summarizing bond covenants or drafting investor reports—without the time and cost required to develop a proprietary solution. On the other hand, a large quant shop opted to build an internal GenAI platform for code assistance and research synthesis, giving them full control over data privacy and customization. The key is aligning the tech decision with scale, budget, and strategic intent. - Implement Strong Governance and Usage Policies
The lack of clear boundaries around GenAI usage can expose firms to legal and compliance risk. One firm introduced an AI policy modeled after its existing code of ethics, explicitly defining what data could be input, what outputs required human review, and who could access internal tools. As part of onboarding, staff were trained on prompt hygiene, data sensitivity, and model limitations—turning policy into practice. - Start with Pilot Programs
Successful firms often begin with low-risk, high-ROI experiments. A long/short equity manager started with a GenAI pilot to automate their monthly investor letters. The results: faster delivery, consistent voice, and more time for the investment team to focus on performance. That success built buy-in and paved the way for broader integration in research and operations. Pilots also help test vendor tools, calibrate expectations, and gather feedback from early users.
Conclusion
In hedge funds, the most successful implementations of GenAI are not splashy—they’re practical. They remove bottlenecks, improve consistency, and free up time for higher-order thinking. The firms leading this shift are blending human insight with machine scale—not betting everything on one or the other.
In short, GenAI isn’t about automation for automation’s sake. It’s about giving the best people better tools. And that’s where the real edge lies.