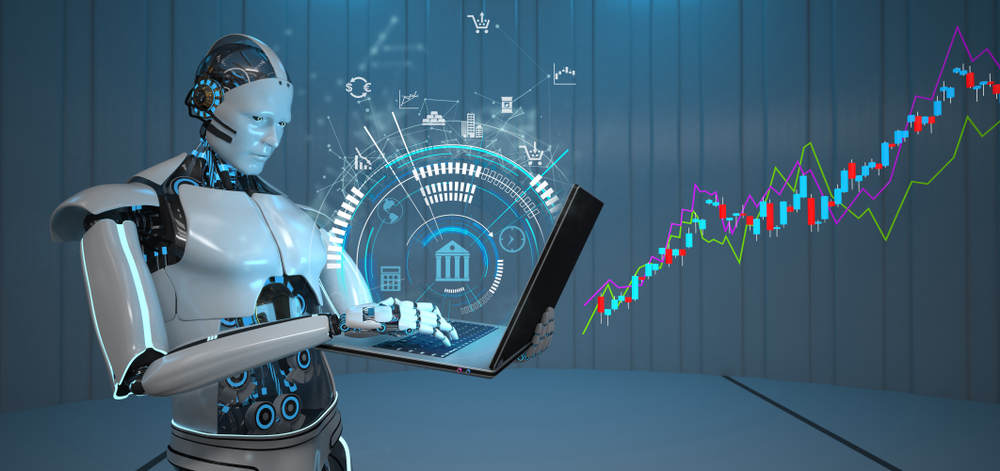
Beyond The AI Hype
Discover how hedge funds attempt to unlock the potential of generative AI in hedge funds with streamlined processes, personalized strategies, and valuable data insights. Explore how AI-driven innovation could revolutionize the industry.
4 min read | Jul 12, 2023
AI is a vast multidisciplinary that seeks to develop computer systems capable of performing tasks that typically require human intelligence, such as understanding natural language, recognizing images, making decisions, and learning from data. It has experienced a remarkable increase in attention over the last decade, following staggering achievements in applications such as image, text and speech recognition, self-driving cars or chess, Go tournaments.
Earlier this year, the release of ChatGPT to the public brought attention to specific subfields within AI, namely Natural Language Processing (“NLP”) and Generative AI (“GAI”). ChatGPT is an NLP models, meaning that it focuses on developing algorithms and models to understand and generate human language, enabling machines to comprehend and generate text-based conversations or written content. In doing the latter, it utilizes generative AI methods, specifically deep learning and language generation models, to produce coherent and contextually relevant responses in natural language.
How is generative AI being used right now by hedge funds?
Investors may not have noticed it, but artificial intelligence (”AI”) is old news in the world of hedge funds. This is probably due to the “AI effect”, where onlookers discount the behavior of an artificial intelligence program by arguing that is not “real” intelligence, thereby tending to redefine AI to mean “AI is anything that has not been done yet”.
Quant hedge funds have been incorporating AI techniques into their investment process for quite some time now, in a variety of applications from leveraging machine learning (“ML”) algorithms to analyze vast amounts of financial data and identify patterns that may lead to trading opportunities, to optimizing portfolio allocations, to executing trades with speed and efficiency. Other “non-quant” hedge funds such as traditional credit managers have been utilizing AI to improve credit analysis and risk management by applying ML to analyze large amounts of financial and non-financial data (incl. data integration and data transformation), in order to assess default risks, predict credit spreads more accurately, or analyze complex credit structures and identify opportunities for relative value trades in credit markets.
What’s relatively new, however, are the new use cases that hedge funds are finding for generative AI applications. For now, improving efficiency by handling mundane and time-consuming grunt work seems to be the immediate one. While not the most sexy, it is nonetheless an important use case that is being rolled out at scale. On the research side for instance, it has to do with improving productivity via the automated management of daily workflows. Generative AI can speed up preliminary analysis by helping to easily digest data such as dense reports, stacks of academic papers on a particular topic, and detecting patterns in data sets. Another example would be to involve generative AI tools in code completion, editing, bug fixes, or simply quicker iterations of trading ideas by typing out ideas in plain English and having ChatGPT write a basic code base to be improved later on. Furthermore, ChatGPT could also assist in automating the grunt work of investor relations, since it can easily explain performance by synthesizing market data and fund returns.
The next frontier for AI at hedge funds: Do More, And Do It Better
Efficiency gains may soon become no longer a differentiator, as increasing standardization and uniformity of processes result in the commoditization of similar capabilities, prompting firms to seek out new value propositions.
Generative AI offers significant potential for financial advisors and investors to leverage conversational text and automatically generate highly customized investment strategies and portfolios. With the explosion of (mostly unstructured) amounts of data, generative AI offers the potential to make that data readily available and manageable for finance across a wide variety of asset classes.
Moreover, generative AI enables rapid and efficient production of data products from underutilized textual data sources. For example, annual reports and filings, like the SEC's 10-Ks, are commonly used for financial statements but contain valuable data that can power product catalogs and comprehensive customer and supply-chain relationship maps across public companies globally. By harnessing generative AI, these data products can be created at a fraction of the cost and effort required by manual or traditional NLP processes.
Annual reports represent just one essential source among the vast amount of unstructured data, estimated to account for 80%-90% of all existing data. Generative AI excels at transforming these extensive repositories of written and spoken word into structured or semi-structured information, empowering investment processes and enhancing interactions with retail investors. Various content sources, such as investment research, investor presentations, earnings call transcripts, broadcast news, interviews, newspapers, trade journals, and websites, can provide valuable targeted intelligence when comprehensively searched and appropriately summarized. This includes insights on pricing trends and consumer preferences for specific products or areas, delivering tangible value to investors.